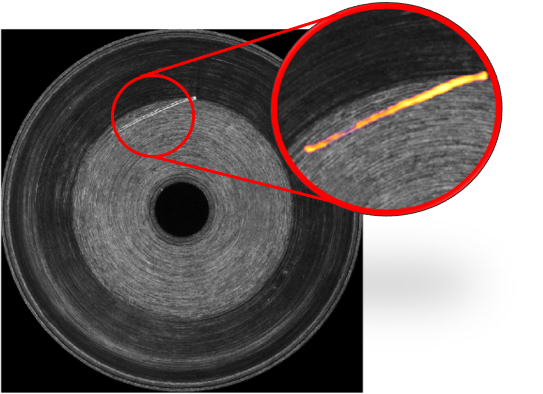
Using Artificial Intelligence for Visual Quality Control
Artificial intelligence (AI) and machine vision (MV) are two powerful technologies that can be used together to improve visual quality control (VQC). AI can be used to learn and identify defects that are difficult or impossible to detect with traditional rule-based Machine Vision systems. This can lead to improved quality and reduced costs.
Here are some of the ways that AI can be used alongside rule-based Machine Vision for Visual Quality Control:
To detect defects that are difficult to define. Some defects are difficult to define in terms of rules. For example, a scratch on a surface may be of different lengths, widths, and depths. Retina AI Vision can be used to learn the patterns of these defects and identify them even if they do not match a specific set of rules.
To detect defects that are rare or intermittent. Some defects may only occur rarely or intermittently. This can make them difficult to detect with traditional Machine Vision systems, which are typically designed to detect defects that occur frequently. Retina Deep Learning technology can be used to learn the patterns of these defects and identify them even if they occur infrequently.
To detect defects in complex environments. Some manufacturing environments are very complex, making it difficult to use traditional MV systems to detect defects. AI can be used to learn the features of these environments and identify defects even if they are hidden or obscured.
In addition to these benefits, Retina AI Vision can also be used to automate Visual Quality Control tasks alongside collaborative robots, which can free up human operators for other tasks. This can lead to improved efficiency and productivity.
However, there are also some challenges to using AI for Visual Quality Control. One challenge is that AI systems need to be trained on large amount of images that contain both good and bad products. This can be a time-consuming and expensive process. Another challenge is that AI systems can be sensitive to changes in the environment, such as lighting or variations in the product. This can make it difficult to maintain the accuracy of the AI system over time.
Luckily, Retina Deep Learning AI Vision is a powerful technology that can be used to improve Visual Quality Control by learning from only a few images of bad products. By combining AI with rule-based MV, we can achieve a higher level of quality and efficiency in the Visual Quality Control processes.
Here are some examples/showcases of how Retina AI Vision is being used for Visual Quality Control in different industries:
Watch & Automotive industry: Retina AI Vision is being used to inspect shiny, metallic and transparent parts for defects, such as scratches, cracks, and dents. This can help to ensure that cars are safe and meet quality standards.
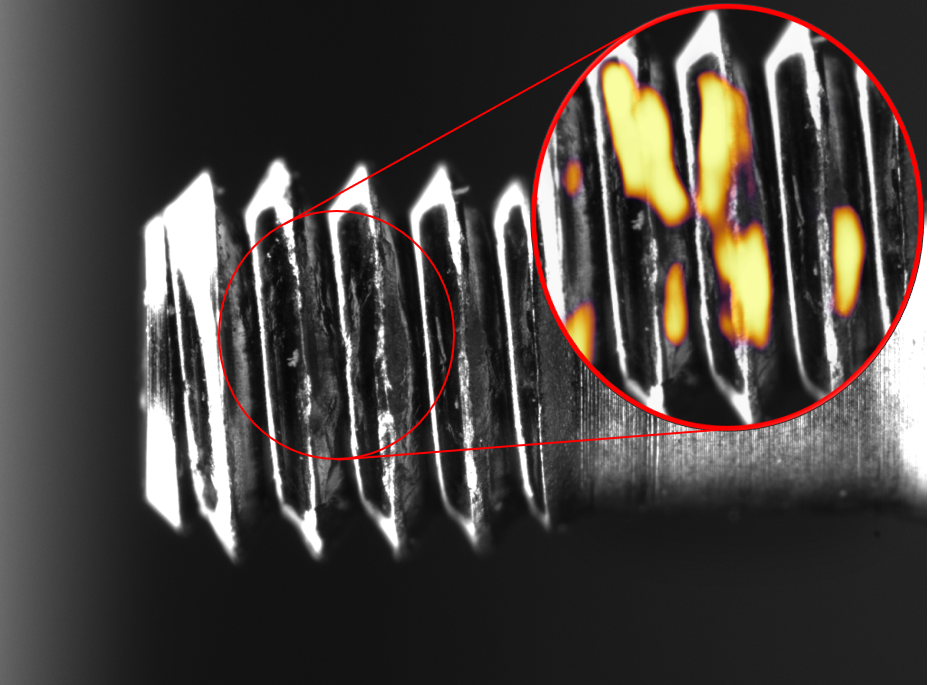
Automating Medical Screws Visual Inspection using Deep Learning Algorithm for Catching Challenging Defects to Enhance Products Quality
Electronics industry: Retina AI Vision is being used to inspect electronic components for defects, such as missing or damaged components. This can help to ensure that electronics products are reliable and work properly.
Food and beverage industry: Retina AI Vision is being used to inspect food and beverage products for defects, such as sealing intigrity, foreign objects and contamination. This can help to ensure that food and beverage products are stored correctly and safe to eat.
Medical devices and Watch industry: Retina AI Vision is being used to inspect surgical devices and watch parts for defects, such as scratches, cracks, and misalignment. This can help to ensure that manufactured products meet quality standards.
As Retina AI Vision technology continues to develop, it is likely that we will see even more innovative Visual Quality Control applications in the future.
Comments are Closed